The new wave of Artificial Intelligence (AI), especially Gen AI including LLMs (Large Language Models), has unlocked opportunities in various industries and verticals. Gen AI is applicable to a variety of use cases democratizing intelligence and empowering people at a large scale. The use cases consist of operational efficiency, productivity gains, improved customer experiences, and new business cases. As a result, many companies are racing to take advantage of the technology and fuel the next generation of innovation and products.
Data Scientists (DS) play a critical role, not only for AI fundamental advancements but also for the successful adoption of the technology on a larger scale. It will be interesting to see how the role, skill sets, and the attributes of Data Scientists (including Data Science function), may evolve in upcoming years. Understanding the change can help us prepare better for the future. For instance, I often get asked a question from data scientists: “if a chatbot can do coding on my behalf, what would I do in the future and what value will I be able to add, and so on”? Data Scientist or Data Science in the entire article is used in a broader sense here, which includes AI/ML Engineers and Scientists and so on. Let’s dig deeper into the question and a few relevant trends:
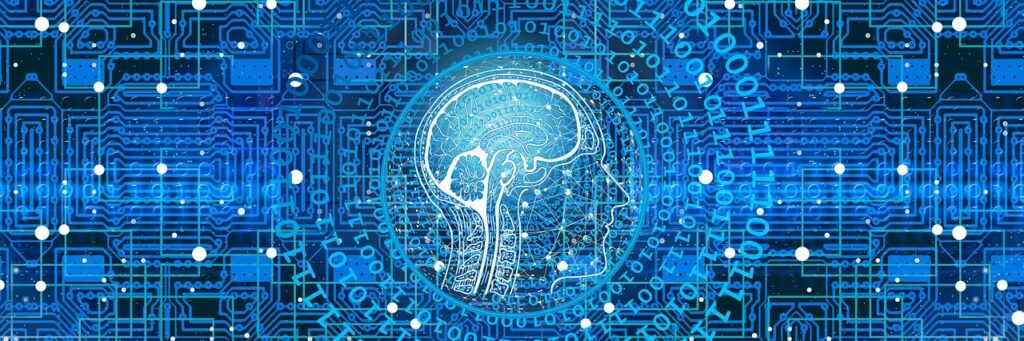
1. Growing Tools and Libraries:
With the growing number of tools and libraries, it is easier and faster to achieve the same business objectives. With a few lines of code, data scientists can create deep learning models in different programming languages. With only a few clicks, users can generate synthetic data and develop computer vision models, which took months a few years back. Furthermore, the same code or even complex code can be instantaneously generated using coding copilots, providing significant productivity gains at task levels [1] or coding levels 50-75% [2]. There are thousands of tools that have been developed and launched in the last year [3]. It means that anyone (including people from other disciplines, or people switching to DS career tracks) can start producing outputs immediately.
The question becomes: how to choose the right tool or a library, even the right configurations, levers, or hyperparameters? As DS projects and products could rely on continuous experiments, it is crucial for data scientists—especially for the ones that are not involved in higher technology readiness levels [4] as opposed to fundamental or core research—to reduce the cycle-time and achieve the business outcomes. To reduce cycle-time and reduce the no. of experiments without compromising quality, it is important that data scientists understand the pros and cons of such tools and algorithms at a deeper level. This includes finding details about the underlying process and technologies that the tool was built upon. Although most DS today are not going to build their models or algorithms from scratch unlike a few years ago, strong DS fundamentals with business consideration will go a long way.
Deeper knowledge and working of public tools and libraries along with strong DS fundamentals will become highly valuable
2. Discipline Expansion:
Advancements in AI and their use cases are huge with high and growing interest from the community in the past 15 months; see Figure 1. Similar trends are observed in this research community as shown in Figure 2. Compared to the advancements, however, the current adoption of AI projects and their delivery success rate as part of core business is still low. To make the AI projects a reality, it is imperative that the AI projects take accountability and ownership in the success of overall business. It goes well beyond creating a model or a proof-of-concepts; it involves enterprise grade development, maintenance, operations, customer experience and support within a safe environment that aligns (or at least not misaligns) with human values
.
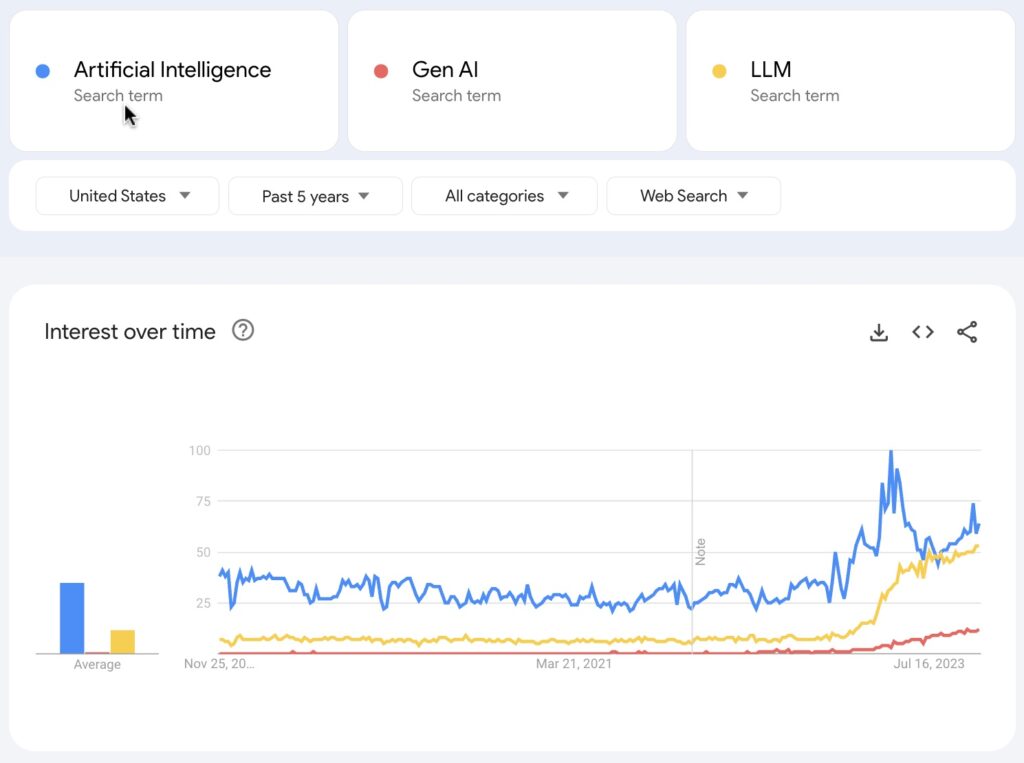
For example, MLOPs emerged as a separate field a few years back for traditional machine learning projects. Furthermore, it is likely that specialized areas and skill sets will be needed given the speed of pace and innovation on multiple fronts. For instance, ML Engineers can be considered as an expert in the model deployment space interacting with engineering functions while Core Data Scientists are specialized in developing new models, exploring uncertain territories working with research. but will be quite challenging for one person to tackle different parts of the ecosystem.
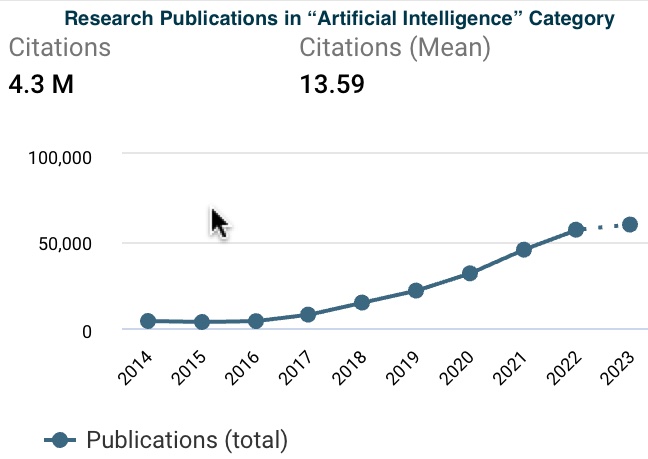
Similar behaviors and trends can be expected within the Gen AI space. In fact, we are already seeing some completely new roles and some specialized roles in the direction such as Inference Manager, Prompt Engineer, Trust and Safety Leads. Given the AI regulation conversations [5] and the new AI bill change [7] as compared to original basic AI guidelines [6], it is reasonable to see new regulation-related roles [8] and functions next year. I would also expect additional roles on governance, cybersecurity (through injection), ethics, responsible data generation, business or domain specific guardrails, and sales.
Product functions such as UI (User Interface), Design, Marketing, Product Management are well defined for SaaS and software applications. However, this is not the case for AI-based applications. Moreover, the UI for existing SaaS applications may substantially change, shifting to natural language interfaces predominantly for certain business functions and industry verticals. As an example, instead of tag-based search, LLMs with vector database solutions have provided a new way to interact (search, retrieve, summarize, etc.) heterogenous documents. People with the understanding of data science and product functions are going to benefit from this transition as new opportunities arise. Rising demand in the AI space will help people from different departments to switch to these roles.
Data Science and AI discipline will evolve significantly to a broader yet diversified scope, becoming increasingly important for core company business depending on the nature of the business.
3. Communication:
It is necessary the stakeholders (internal, external, cross-functional) comprehend and value the capabilities, investments, and working of AI systems because of:
- The nature of the DS work and projects. For example, AI may not always provide accurate results, such as missing an object or dog in a picture, although there is tremendous amount of work in progress to improve the performance. Similarly, Gen AI (including LLMs) may lead to inconsistencies or lack with replication issues. In the same case, it may not be straight-forward to demonstrate the value in the short-run. Different frameworks such as ROI-based, OKRs, etc. can be used depending on the audience.
- Change in the DS landscape. As mentioned earlier, 1000s of tools are being developed requiring new and specialized skill sets and investments. At the same time, because of the talent gap and growing demand, it is easier to switch to a DS career track and develop quick PoCs. However, bringing such projects/products to fruition requires buy-in from multiple teams not only internal departments but also end-users to address their concerns at a deeper level.
Gen AI and LLM is relatively still new for most companies in terms of its usage. Most companies (in low single digit % numbers) don’t have production grade workload for external users. Until AI becomes mainstream, it is beneficial to make sure that the end-customers are equipped with the right tools to handle their internal stakeholders’ questions as this is new to most of us.
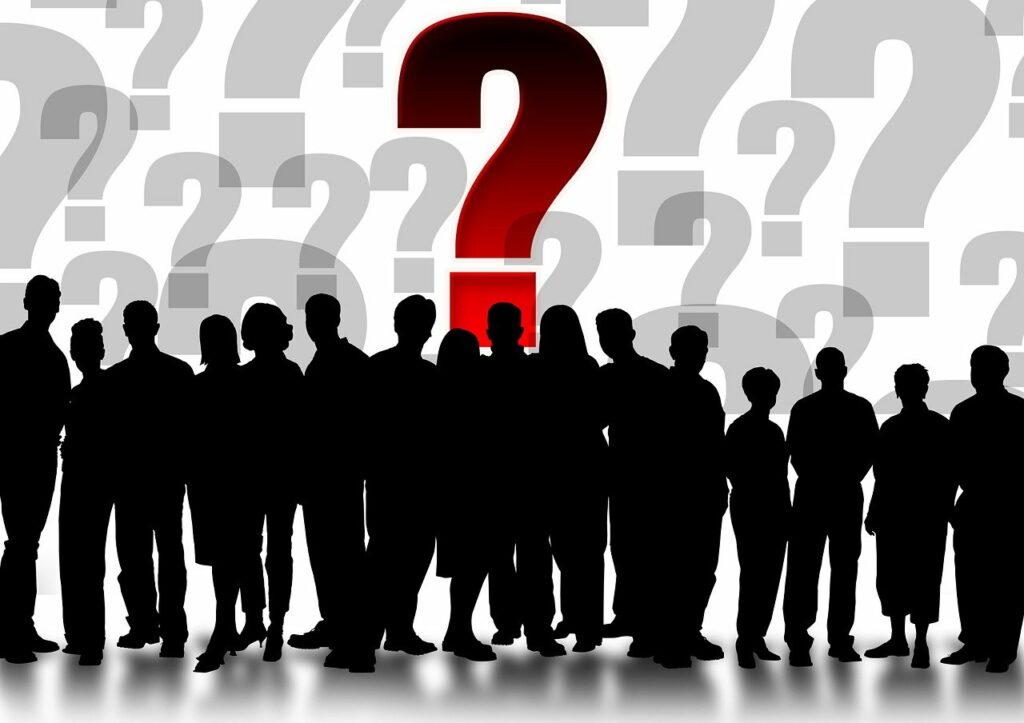
Understanding the business at appropriate levels, ability to communicate with the different stakeholders, and setting right expectation will be differentiator for data scientists.
As Data Science and AI offerings expand, this is a growth opportunity for data scientists to refine or set a new career path in the direction that aligns with their long-term interests and preferences. Despite many unknowns and uncertainties, it will be exciting to see how all of us contribute to this rapidly changing field while having a positive impact on society.
Keep learning and keep moving forward!!
References
- Dell’Acqua, Fabrizio, et al. “Navigating the jagged technological frontier: Field experimental evidence of the effects of AI on knowledge worker productivity and quality.” Harvard Business School Technology & Operations Mgt. Unit Working Paper 24-013 (2023).
- Meyer, André N., et al. “Software developers’ perceptions of productivity.” Proceedings of the 22nd ACM SIGSOFT International Symposium on Foundations of Software Engineering. 2014.
- “The Best New Products in Tech.” Product Hunt, www.producthunt.com/. Accessed 19 Nov. 2023.
- “Technology Readiness Levels.” NASA, NASA, 27 Sept. 2023, www.nasa.gov/directorates/somd/space-communications-navigation-program/technology-readiness-levels
- “Blueprint for an AI Bill of Rights.” The White House, The United States Government, 16 Mar. 2023, www.whitehouse.gov/ostp/ai-bill-of-rights/.
- “Executive Order on the Safe, Secure, and Trustworthy Development and Use of Artificial Intelligence.” The White House, The United States Government, 30 Oct. 2023, www.whitehouse.gov/briefing-room/presidential-actions/2023/10/30/executive-order-on-the-safe-secure-and-trustworthy-development-and-use-of-artificial-intelligence/.
- “AI Regulation Is Coming- What Is the Likely Outcome?” CSIS, www.csis.org/blogs/strategic-technologies-blog/ai-regulation-coming-what-likely-outcome. Accessed 19 Nov. 2023.
* AI (Gen AI, LLM, etc.) was NOT used in creating any content of this articles*
**This article does not cover any common trends in AI, it will be covered in a separate article**