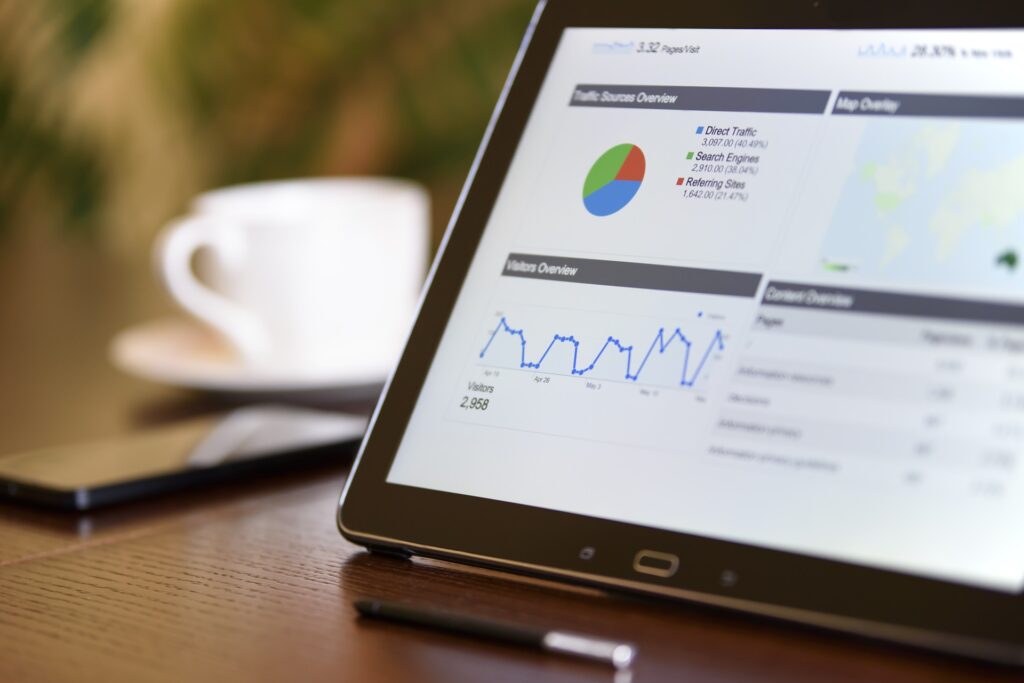
We all know and understand the value of data. Data can help businesses make better, faster, and smarter decisions. We can use the data for daily operations and long-term strategic planning. Data-driven systems are essential part of an organization, as they can drive many business cases ranging from operational efficiency to product improvement to customer experience (Kramer, 2021). For example, data collected from smart thermostats inside homes can be utilized to understand occupants’ behavior and preferences, such as what time people get up vs. when they go to sleep. This behavior can be utilized further to optimize energy operations inside homes or commercial buildings (Goyal, 2021). Many companies plan to integrate (if already not incorporated) data-driven intelligence into their organization whether it involves product, process, or a decision. One of the major requirements in achieving the vision of data-driven intelligence is harvesting “good” data.
What happens when the data is “bad”?
If the data is bad, can we use it in a meaningful manner? Can we develop an AI/ML (Artificial Intelligence/Machine Learning) system reliant on such data? Can we trust the decisions? You might have heard a common phrase “Garbage in, garbage out”. In this context, it means that the outcome is unreliable and untrustworthy if the input data is “bad”. Is this really the case?
Answers to these questions depends on two main factors: 1) attributes of the data such as accuracy, reliability, definition, scope adherence, sampling intervals, and 2) the purpose and intent of data usage.
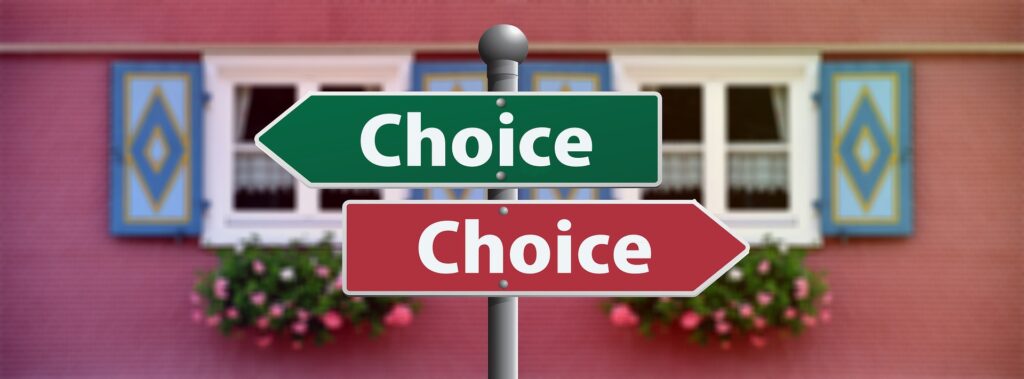
Let’s take an example in an ecommerce business, where users collaborate to buy and sell products in an online marketplace. Suppose a certain item (e.g., a watch from a new seller) or a class of items are not accurately rated on the platform. The current rating of the watch is 3 stars, which is not the true rating. There can be several reasons why there is a discrepancy between the true rating and the displayed rating. For instance, the rating can be inaccurately calculated by the ML techniques through text-reviews, or the platform is designed inappropriately as it encourages users to rate incorrectly (3 stars instead of 4 or 5 stars). In any of these cases, the data does not reflect the true rating of the product.
One might call the data bad, but is the data completely useless? If the same so-called “bad” ratings are being displayed publicly, the ratings can affect the other (potential) buyers and future sales. Buyers may perceive the watch as bad, and decide not to buy the product resulting in lost sales. The buyer may be shopping around and switch to a different website. If the seller is experiencing declining sales, the seller may leave the marketplace and join a competitor marketplace. This behavior influences the overall usability and effectiveness of the platform. Regardless of the true rating (or the accuracy) of the product, we can use the data in several ways such as understanding the relationship(s) between the customer perception and sales, or other intermediate steps in the funnel, including seller churn/retention.
The short answer is: Yes, we can utilize the data, but we have to understand the business problem in addition to the limitations and context in/for which the data was generated.
References
Kramer, A. (2021). Embracing Data Analytics for More Strategic Value. Harvard business review Analytical Services.
Goyal, S. (2021). Advanced Controls for Intelligent Buildings: A Holistic Approach for Successful Businesses (1st ed.). CRC Press. https://doi.org/10.1201/9781003176589
Any thoughts or feedback?